Annotation in Machine Learning: A Comprehensive Guide for Businesses
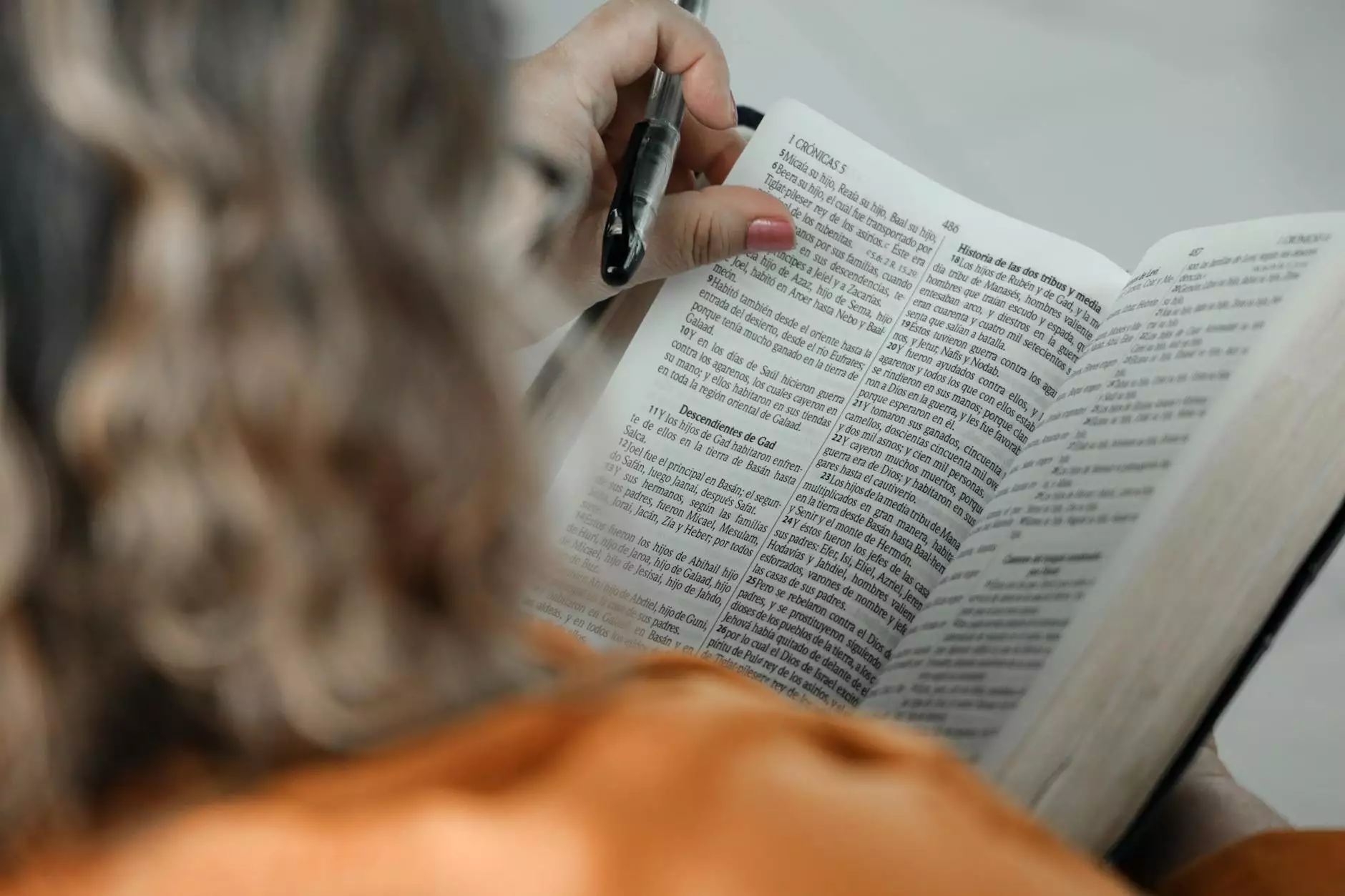
Annotation in machine learning plays an essential role in the development of intelligent systems. It involves the process of labeling data, which is a crucial step for training machine learning models to understand and interpret complex information. This practice is not only relevant in tech industries but also has significant applications in various business sectors, including home services and locksmith services, like those provided by KeyMakr.
What is Annotation in Machine Learning?
In the realm of machine learning, annotation refers to the act of tagging or labeling data so that it can be understood by algorithms. This data can take various forms, such as images, text, audio, or video. Proper annotation enables models to learn patterns, classify inputs, and make predictions. Here’s a breakdown of different types of data annotation:
- Image Annotation: Involves marking objects within images which is particularly useful for object detection in computer vision.
- Text Annotation: Includes tagging parts of speech in text, sentiments, or categorizing information for natural language processing.
- Audio Annotation: Consists of transcribing spoken language into text or labeling sounds.
- Video Annotation: Involves drawing bounding boxes around moving objects or labeling specific actions in videos.
The Importance of Annotation in Machine Learning
Annotation is vital for several reasons:
- Accuracy in Model Predictions: Well-annotated data enhances the accuracy of machine learning models. Models trained on quality data perform better in real-world applications.
- Facilitating Understanding: Annotation helps machines understand context and nuances in data, which is particularly important in fields like natural language processing.
- Improving User Experience: Properly annotated data enables businesses to develop systems that can interact intuitively with users, enhancing customer satisfaction.
- Scalability: With the right annotations, systems can learn from new data and scale efficiently without starting from scratch.
Use Cases of Annotation in Machine Learning
Multiple industries leverage annotations for improved processes and services. Below are some noteworthy applications:
1. Healthcare
In healthcare, machine learning models trained on annotated medical images can assist in diagnosing diseases, streamlining patient care, and predicting patient outcomes.
2. Automotive
Self-driving cars utilize a plethora of annotated data, including images and video, to recognize and navigate different environments and obstacles.
3. E-commerce
Online retailers employ text annotation to enhance search functionalities, ensuring that customers can find products quickly and easily through intelligent recommendations.
4. Home Services
Businesses, such as KeyMakr, can utilize annotated data to optimize services like locksmithing. For instance, their systems can learn from annotated images and user inquiries to provide faster and more accurate service.
Challenges in Annotation
While annotation is essential, it is not without challenges:
- Time-consuming: The process of annotating data can be labor-intensive and requires significant time investment.
- Quality Control: Ensuring the accuracy of annotations is critical. Inaccurate labeling can lead to poor model performance.
- Cost: Hiring skilled annotators or using advanced annotation tools can be costly for businesses.
Best Practices for Effective Annotation
To overcome these challenges, businesses can adopt several best practices:
- Define Annotation Guidelines: Establish clear rules and standards for annotators to maintain consistency in labeling.
- Use Annotation Tools: Employ specialized software tools that can streamline the annotation process and improve efficiency.
- Incorporate Feedback Loops: Regularly review annotations and model performance to make necessary adjustments.
- Invest in Training: Provide training for annotators to enhance their understanding of the subject matter, ensuring high-quality annotations.
How KeyMakr Utilizes Annotation in Machine Learning
As a prominent player in the home services and locksmiths industry, KeyMakr uses annotation in machine learning to improve their service offerings effectively. Here’s how:
1. Enhanced Customer Support
By implementing chatbots trained with annotated data, KeyMakr can handle inquiries efficiently, providing immediate assistance and significantly enhancing customer satisfaction.
2. Efficient Resource Management
Annotations allow KeyMakr to analyze service requests and customer feedback to allocate resources effectively, ensuring that they can meet demand without overextending themselves.
3. Predictive Maintenance
Using annotated historical data of service calls, KeyMakr can predict when locks or security systems are likely to fail, enabling them to offer preventive maintenance services.
4. Marketing Insights
By analyzing customer interaction data that has been annotated, KeyMakr can identify trends and customer preferences, allowing for targeted marketing strategies that resonate with their audience.
Conclusion
In conclusion, annotation in machine learning is a powerful tool that can transform data into actionable insights. As businesses continue to adopt machine learning technologies, understanding the importance of effective annotation will be imperative. Companies like KeyMakr are already reaping the benefits, enhancing their home services and locksmithing solutions through improved data practices.
By focusing on quality annotation, organizations across various sectors can unlock new opportunities, drive innovation, and ultimately deliver superior value to their customers.
For more insights into machine learning and home services, visit KeyMakr.